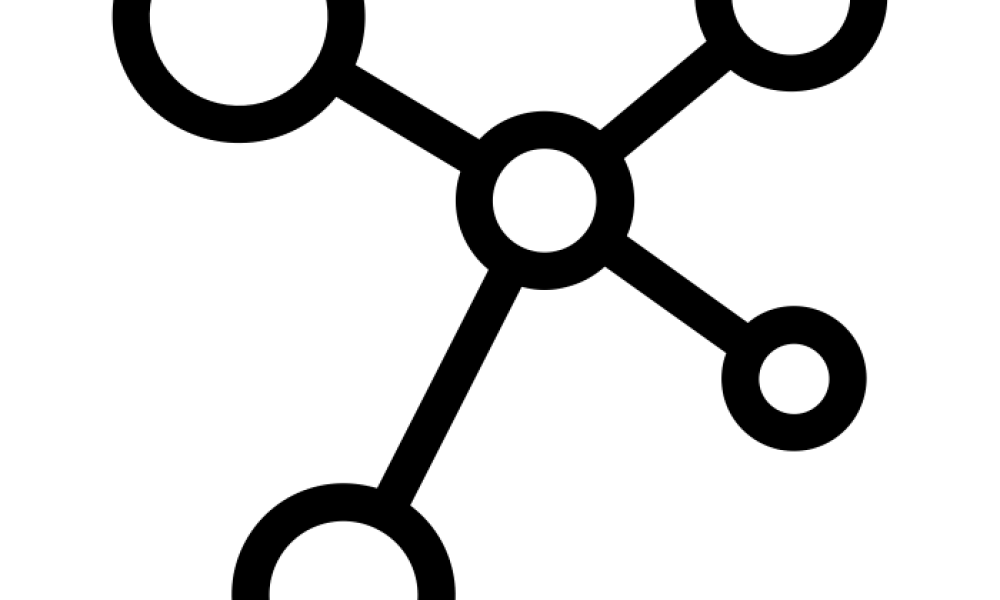
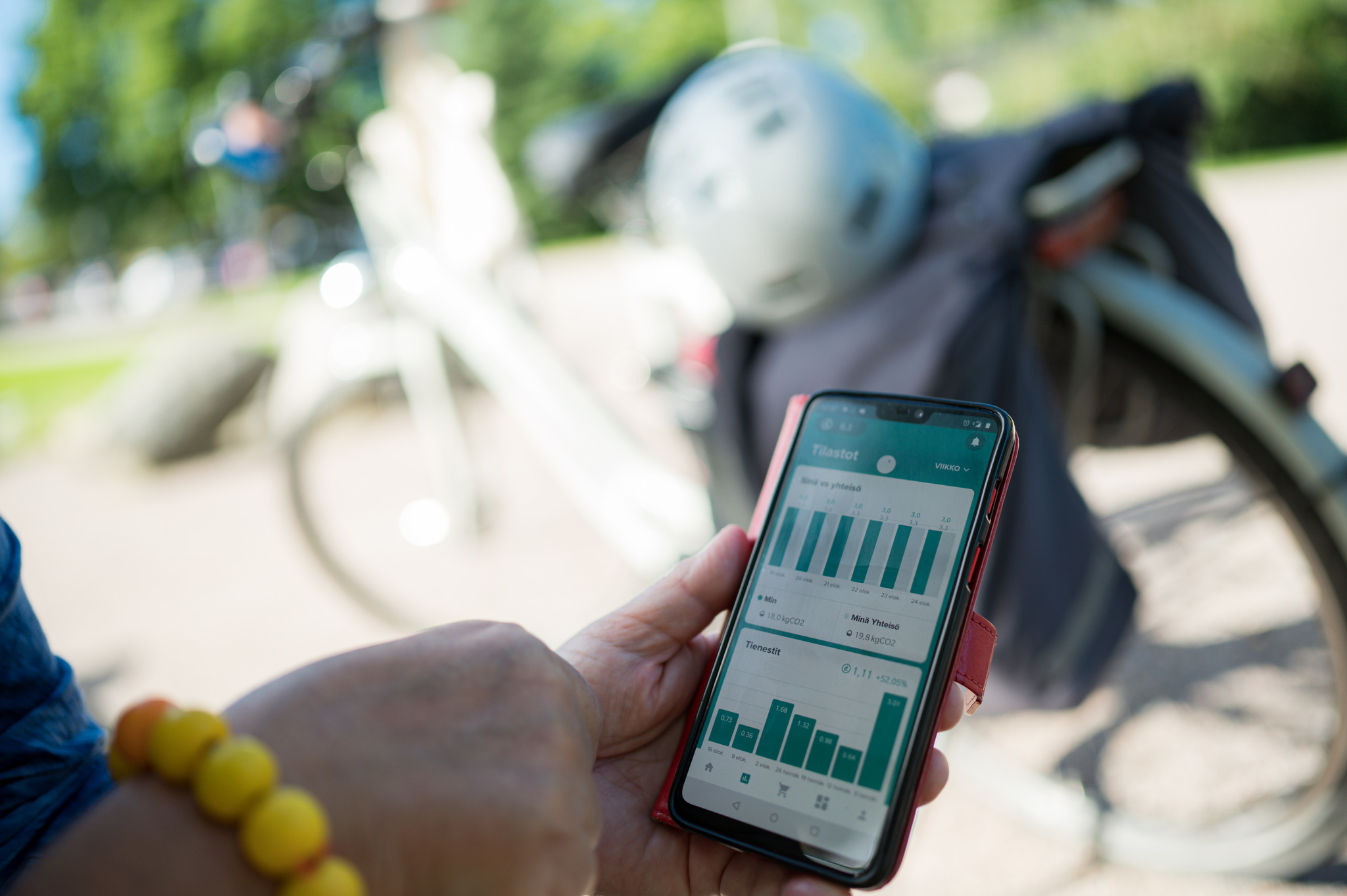
The vision of a city in which Mobility as a Service (MaaS) offers citizens a ‘genuine alternative to the private car[1]’ is one towards which city planners are currently striving. There are generally two parts to the vision (put into practice by Ghent for example). First, reducing reliance on private cars through urban redesign (Barcelona’s superblocks, Paris’s 15-minute neighbourhood/city, Ghent’s ‘car free’ centre, etc.) and rethinking work, such as making more use of teleworking. Second, putting in place alternative transport options, including public and/or private options to create a multimodal network, in which transport is provided as a service. Of course, shifting away from the private car, which remains irresistibly convenient in cities designed for cars, is a challenge. Studies conducted in the United States show that the use of public transport drops by up to 90 percent when passengers must walk more than half a mile to the nearest transit stop[2]. To appeal, the overall transport offer, whether public or private services, must therefore offer complete and convenient journeys. In turn, this means developing an integrated network, in which the ‘first mile/last mile’ can be serviced by emerging mobility options, from shared EVs to various forms of micro-mobility. The key to making MaaS work is network integration (offering seamless journeys) and this in turn requires that information – i.e., data - (e.g., routes, schedules, wayfinding apps[3]) is made available to citizens.
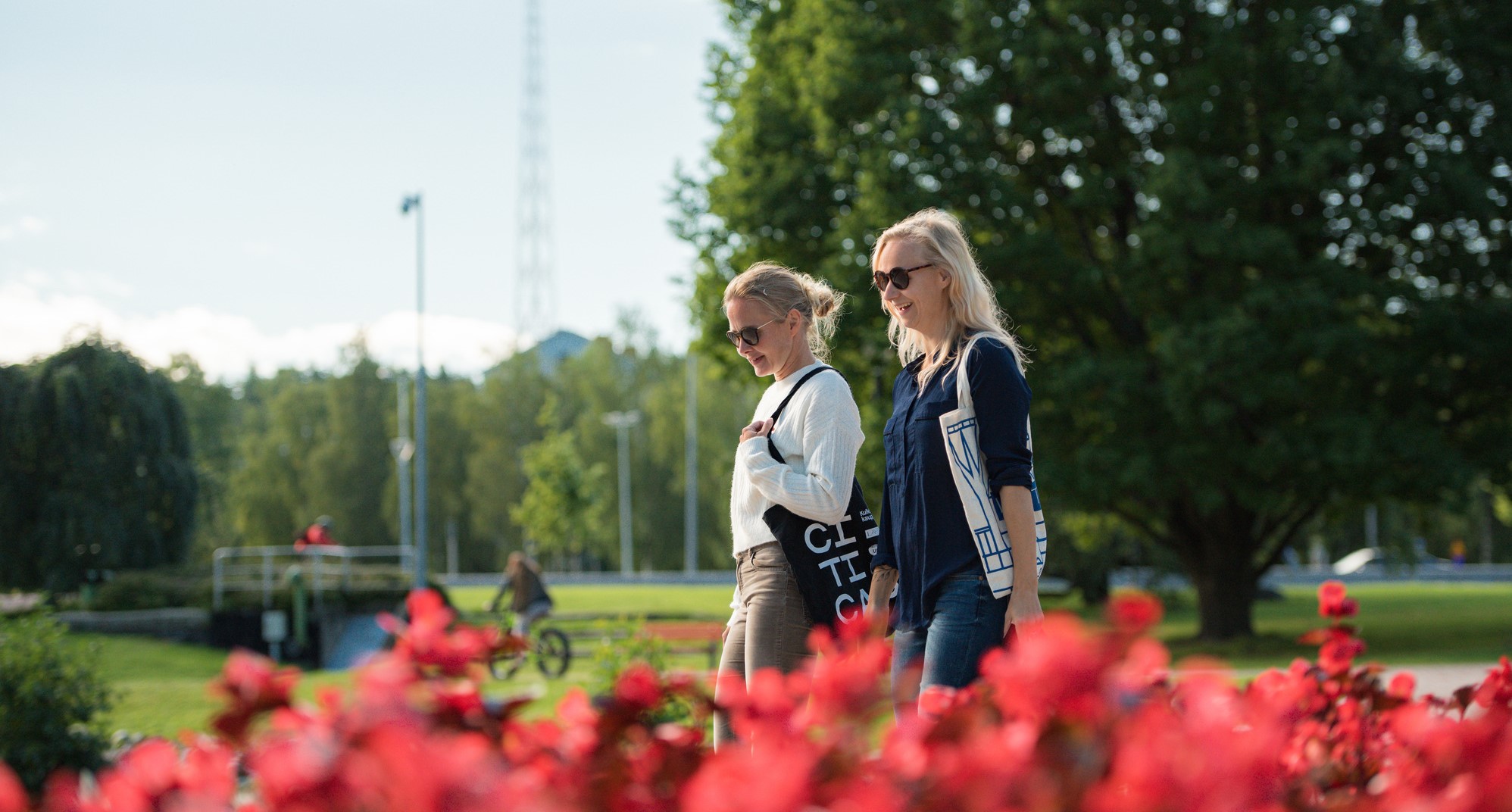
Data, of course, has always been essential for strategic mobility planning and policy. What is different today is the confluence of new transport modes, the utility of ‘big data’ as strategic intelligence, the emergence of mobility data analytics, and the ubiquity of mobile devices. Intelligent mobility, defined as ‘moving people and goods around in an easier, more efficient and more environmentally-friendly way[4]’ is a complex coordination challenge. From developing the vision to building partnerships, from designing a network to delivering MaaS services, the fuel needed to make a reality of intelligent mobility, both materially and digitally, is data. The UK Catapult initiative, for example, argued in 2015 that data would become a key driver of future transport planning[5]. Governments[6] [7] and cities, including UIA cities, have begun to capitalise on the potential of data as a driver of new mobility services, including placing their data in the open domain, as Ghent has, for example. The intelligent mobility market is rapidly expanding, with an estimated global value of 900 billion by 2025[8]. Industry stakeholders, such as the World Business Council for Sustainable Development (WBCSD), also believe data sharing to be crucial to realising the vision of multimodal transport and has published guidance on the principles of data sharing. The International Road Federation (IRF) echoes this view[9]. In the EU the European Commission has also produced expert reports[10] and supported numerous projects in this area (SMILE[11], PETRA, CIVITAS[12], UIA Cities, etc.).
The sources and uses of data
Mobility data can come from conventional counters or surveys[1]. Big data, however, brings with it many new sources which offer exploitable location and travel data. These include smartphones, social media[2], TomTom, Vaze, Google, or other geolocation-based services. Other new sources include the public or private transport providers responsible for car-sharing stations, e-car charging points, bike-sharing stations, or car-pooling services. Data on activities such as cycling and walking are easier to collect than in the past, etc.
All of this new data can be sorted into categories, including, at least:
- Cartographic, weather data;
- Personal location data;
- Data on: transport schedules, fares and prices, network disruptions, planned events, real-time network capacity for people, vehicles & goods;
- Vehicle location data;
- Performance evaluation data from service users and non-users; third party service usage data; and payment/transaction data[3];
- Information about disabled access, lifts, etc. for improved inclusivity[4].
[1] Origin-destination studies, traffic counters, etc.
[2] Data and digital systems for UK transport: change and its implications, Dr Caitlin D Cottrill, UK Govt Office for Science, Future of Mobility, Evidence Review, Dec 2018.
[3] The Catapult Data Revolution, Investigation into the data required to support and drive intelligent mobility, CATAPULT, March 2015, page 5.
[4] Urban Mobility Company, The Urban Mobility Daily. Why Open Data is Critical to the Future of MaaS, Sandra Witzel, 23/09/2020.
Data is used to inform (short and long-term) infrastructure/service planning and day-to-day traffic management by identifying patterns of mobility demand and by helping planners to understand a transport network’s traffic flows and status. Data analytics can reveal unsuspected links and connections in mobility patterns, it can match weather patterns with traffic fluctuations, and can predict the traffic flows likely to be generated by changes in the weather or by large-scale events, such as a football game or a concert. Data analytics can give insights into accidents or predict how voyagers might be affected by them. It can also predict air quality, etc.
Data is the essential ingredient in MaaS apps aimed at citizens although technological developments are also key to enabling these information services. In this regard, API[1] technology is making it easier to merge information from different mobility services and modes of transport and other relevant sources (weather, travel alerts, etc.).
The ‘true potential’ of data to support mobility is still ‘largely unknown’[2]. Nonetheless, the role of data in the emerging transport landscape is being hailed as a ‘revolution’[3]. ICT enabling technologies are in general enabling unprecedented B2B, B2C, and C2C services. Industry experts believe that, in the field of mobility, open data (OD) platforms will be indispensable to intelligent multimodal transport and in particular MaaS[4], placing importance - for cities - on establishing mechanisms for data sharing and ensuring their mastery of ICT enabling technologies, for example.
Data platforms (aka portals) can support applications which can then easily make data available for specific uses, thus providing value to the city and voyagers[5]. Apps can include dynamic journey planning (optimum or ‘most enjoyable’ route generation) for example and can reduce travel times for voyagers. As part of an integrated approach, it is believed that data has the potential to disrupt the car-dominated transport model and to help reinvent transport services. For many industry observers, data is set to become ‘nervous system’ of future sustainable urban mobility systems[6], enabling cities, among others, to:
- Roll out Mobility as a Service (MaaS) with single ticket journeys across multiple modes;
- Contribute to city planning (land use), and generally better inform city development policies;
- Optimise real-time traffic and parking management;
- Optimise the regulation, operation, and control of smart city infrastructure (multimodal platforms, public transport, including avoiding congestion caused by road works, etc.);
- Drive a modal shift in commuting/traveling towards lower-impact vehicles/alternative soft modes, thereby reducing pollution, pollution peaks, and increasing road safety;
- Help cities to deploy new access rules for private and public transportation and identify new streams of revenue;
- Enable the future integration of connected self-driving vehicles in multi-modal networks;
- Help to combat the spread of diseases (pandemic response);
- Service evaluation. As travel experience is becoming a decisive factor in citizens’ decisions to opt for one transport mode over another, it is important that cities gather user satisfaction data. Key Performance Indicators (KPIs) can support a people-centred approach to mobility. For example, the SMP 2.0 Sustainable Mobility Indicator set, developed by the WBCSD, includes soft indicators on journey experience, such as on ‘comfort and pleasure’. In another example, the EU the Urban Agenda (2019) Partnership on Urban Mobility – Action 3 – highlights the need for a new ‘simple walking indicator’ for improving urban accessibility. Rather than simply focus on journey time or walking distance to the nearest bus stop, consumer satisfaction should also be measured. As the collection of qualitative data is easy with sensors and smart phone applications, KPI indicators can generate feed-back on travel experience. Such feedback also helps service providers to understand the reasons why customers may be unhappy with a service, enabling them to address issues and improve their services.
In many EU cities, the two types of data gathering methodologies, conventional and ‘new data’, are used in parallel. While the future of large-scale representative surveys and travel counts is uncertain, the two methodologies can be complementary. In many cases, the digitally sourced data can fill in the time gaps for conventional survey methods or provide more frequent feedback, while traditional counting methods can verify and refine data collected through sensors by providing the demographic background behind this data (automatically generated data sets may miss invisible users). One of the biggest challenges facing cities is how and to what extent to switch from conventional methods to work with digitally created data (real time/ big data, sensor based). Is it possible to fully rely on new data analytics methods or will it only provide information on specific segments of travel behaviour?
Data governance, i.e., designing surveys and selecting the right mix of survey methods and data acquisition for a project (what data, what for?) is part of a wider mobility planning process, which implies that cities must possess the relevant expertise and planning capability. Veeneman et al (2018)[7] call this a ‘governance challenge’. Cities’ ability to work with digital data varies and to master big data many will need to build capacity and develop data governance strategies, with cross-departmental working at a minimum. Air quality (CO2), travel times, user satisfaction, road safety, congestion, land use, etc., are all expressed through data.
Working with big data (new sources and types of data) may imply infrastructure/equipment costs, including investment in sensors and/or software, as well as an ability to enter into agreements with data owners. All of this may require the creation of in-house teams with the relevant technical skills, as well as the presence of and ability to comply with legal frameworks governing data gathering, storage, and use, etc.
For cities such as Lahti and Toulouse, both implementing UIA mobility projects, a reliable data management system is an essential first step. This implies:
- establishing data gathering systems (conventional, in-house, bought in, big data, etc.);
- coordinating the different streams of information;
- building up models from the analysis of very large amounts of complex and geographically distributed sources of data (citizens, traffic, vehicles, city infrastructures, IoT devices, etc.) collected in heterogeneous formats (need for metadata, linked to the city’s goals). Then, visualising the information in a format which enhances understanding and allows better decision-making, including enabling stakeholders to (1) raise useful questions (exploiting the synergies of cross-departmental and external collaboration) and (2) build a shared vision of mobility needs and solutions.
Many transport organisations are now routinely providing data that enables Mobility-as-a-Service applications to provide real-time information to customers. The data can also be used to create centralised data platforms which can then support communication between operators and voyagers via apps. There is a no definitive checklist on the uses of data, and, with reference to the content of projects implemented by the five UIA cities, specific uses tend to be linked to specific local objectives.
Table 1 (below) presents insights from the 5 UIA cities on the emerging use of new data techniques (greatest challenges/opportunities/data sets used/data control/in-house processing/processing needs).
[1] Application Programming Interface (API) enables separate systems to interact and share data.
[2] World Business Council for Sustainable Development (WBCSD), Enabling data sharing, Emerging principles for transforming urban mobility, Jan. 2020.
[3] Catapult, Technology Strategy, 2016 for Intelligent Mobility.
[4] Urban Mobility Company, The Urban Mobility Daily. Why Open Data is Critical to the Future of MaaS, Sandra Witzel, 23/09/2020.
[5] Veeneman et al (2018) PETRA, Governance as a key success factor for big data solutions in mobility, Research In Transportation Economics, Elsevier, Vol.69, 2018.
[6] International Road Federation, Data Sharing for Urban Mobility, July 2020. News article.
[7] Veeneman et al (2018) PETRA, Governance as a key success factor for big data solutions in mobility, Research In Transportation Economics, Elsevier, Vol.69, 2018.
Overview of Survey Responses by city (a general discussion follows)
A survey questionnaire asked the five IA cities to provide feedback on the benefits and difficulties of using data and the types of data they are using in their projects/city mobility planning. The following paragraphs summarise, for each city, the key points in their feedback.
To know more about this project, click here!
In addition to biannual traffic and speed surveys, Albertslund is seeking to integrate data from new sources, including automatic sources, such as sensors in traffic lights and is also testing drones to obtain data. This data is helping the city with its different mobility goals including reducing congestion, improving maintenance and road safety, and reducing pollution and CO2 emissions. Albertslund also conducts surveys to obtain data from citizens, especially from schoolchildren and cyclists and the LINC project uses a co-creation approach which will exploit user feedback during trials to ensure it meets their needs (co-creation). The local DOLL[1] initiative should also enhance data gathering capabilities there are (ongoing tests for dynamic traffic detection, intelligent parking, and connected autonomous vehicles).
Albertslund established a data governance structure across all its city departments, including mobility, in order to ensure full (public) control of how data is monitored and stored. The city is currently working on its data management and reports challenges of a technical nature relating to the switch from using conventional data to using big data. The city reports that it needs more skills in data management, where there is current a capacity gap. However, it does make its data available to third-party service providers.
In addition to this, and in the context of establishing PPP arrangements involving private companies, Albertslund suggests that companies could also bring in not only data, but also alternative sources of funding through their own investments and that, in their view, there is a need for good practice models in this area. Albertslund suggested the example of investment in e-charging stations, for which it is currently envisaging a PPP collaborative format.
[1] Danish Outdoor Living Lab
To know more about this project, click here!
Ghent collects conventional data through a triennial survey for modal split data and uses ad hoc surveys. It also gathers multiple mode and real-time data, including buying in data from private suppliers, which is exemplified in Ghent’s UIA project. The Ghent TMaaS project is centred on the use of data and data management/use technologies to enhance traffic management through the creation of an affordable ‘traffic centre’ data platform (TMaaS stands for Traffic Management as a Service). The platform automatically monitors traffic and mobility for the city of Ghent, providing traffic operators and end-users insight in mobility and notifying them with useful transit related data. It can incorporate different data sources to achieve this. From a data point of view it is not just the incorporation of data sets which characterises the project, but the essential wide partnership needed to deliver the platform, which has required Ghent to prepare agreements with companies, which cover data use.
Ghent has also begun to use feedback from citizens. As in Lahti, this has enabled Ghent to better understand usage and make improvements. As in Lahti the feedback comes from cyclists for the purpose of improving routes. In Ghent, the feedback informs route planners, which go one better than standard planners in that they identify the qualitatively ‘better’ routes rather than the shortest identified by private services. The approach takes a more psychologically sophisticated approach to understanding choices between modes and to changing behaviours.
Ghent reports several types of data management challenge. These include: multiple data sources, large volumes of data, a lack of meta data (i.e., consistent data categories), leading to data storage problems (data lake), and data security issues. In terms of delivering transport policy, Ghent uses a mobility company, although it also indicates there remain cross-departmental barriers hindering data sharing.
To know more about this project, click here!
Lahti’s CitiCAP project has different strands to its sustainable mobility goals, with mobility data at the heart of design and delivery. The general purpose of the data is to inform and incentivise the use of green mobility and reduce the use of private cars. CitiCAP is part of the city’s ambitious goal that by 2030 more than 50% of all trips will be made using sustainable modes of transport.
Lahti’s PCT Scheme uses data on CO2 emissions added to financial incentives to encourage citizens to switch to green modes. Moreover, usage/demand data is gathered and is indeed generated, for example on spatial aspects of green mobility, with this data – knowledge – then used to inform the city’s SUMP process. SUMP development is a participative approach, which encourages stakeholder buy-in and enhances the prospects of success by tailoring solutions to needs. Lahti’s PCT scheme uses this approach ‒ so data gathering, data generation, and communication informed by relevant data, is at the heart of this approach (again, as with Albertslund LINC project we can see how a data flow is central to co-creation).
In terms of data gathering, Lahti’s use of new data techniques can be seen against the background of a conventional approach based on 6 yearly surveys. Like the other UIA city projects, Lahti reports that the switch from conventional to new data brings with it a series of data management challenges. These include the sheer volume of different sources and types of data available, which is technically difficult to exploit, although new data sources can be less expensive and provide higher frequency insights than conventional methods. The new data is also indispensable to developing green policy alternatives because it reveals change in use and can reveal user preferences (are buses comfortable, for example), and therefore provides useful data about demand dynamics. Another generic issue reported by Lahti, and which is also present in several of the UIA projects (Ghent, Toulouse), is that data management, from collection to visualisation, requires specific technical skill-sets.
The extensive incorporation of data in mobility solutions is a case in point. Developing online applications (Journey planners, PCT, and so on) implies integrating associated ICT technologies such as API, which enables devices to communicate. Journey planners inform citizens about the multimodal services available to them, while PCT informs and incentivises a modal shift. Both of these strategies require data and sophisticated technologies to communicate that data.
To help in the development of mobility apps, Lahti is moving towards an open data policy and is seeking to integrate new sources of data (such as real-time data from traffic light sensors or from buses), all of which implies mastering API technology. The city is requiring service providers to share their data too, as a condition for market access (e.g., e-scooters). And the city makes its data available to service providers, including Google’s Transit Trip Planner, a national app, + others (e.g., Moovit, Nysse, TripGo).
In addition, in some cases, such as the PCT Scheme, which collects data from a range of transport modes, there can be specific management challenges, such as GDPR constraints, which imply a need for legal expertise.
To know more about this project, click here!
To know more about this project, click here!
Szeged’s conventional mobility data collection includes a 3-year modal split survey, with a focus on public transport. Szeged has attempted to control high-levels of private car use, and the pollution/CO2 this generates, by investing in its public transport and cycling infrastructures to give citizens access to attractive alternative sustainable mobility options. To convince citizens to board a tram or a bus or to use a bicycle, the city has developed a set of outreach approaches, targeting employers in particular, as they have the power to influence commuting dynamics. The city believes that, along with improved services, if stakeholders and users better understand mobility options, then they can reduce the entrenched reliance on private cars. Until SASMob, efforts to increase cycling had had some success, albeit at the expense of public transport.
The data-based approach under SASMob involves mobility tracking, using smartphones, to better understand mobility needs, which then can inform bespoke solutions. This tracking has been possible thanks to the participation of the Szeged Technical University, which has patented a novel workaround to the issue of data privacy (GDPR), essentially anonymising the data collected. This is important because understanding flows and therefore the needs of specific populations is fundamental to the strategy of targeting these population with behavioural change schemes. The approach is epitomised by the SASMob Pledge, which identifies sustainable mobility solutions for employees, with employer support, to reduce commuting. The approach is interesting because it does not see users as a homogenous group, but as distinctive groups with distinctive needs, which can be targeted.
Szeged is seeking to integrate new forms of data and types of data to enhance its mobility policies. This includes gathering data on public transport use ‒ with a view to optimising service provision and quality (comfort), including asking citizens to report any vandalism. It also includes real-time tracking to analyse, for example, congestion caused by refuse vehicles. The data issues Szeged sees as challenging include: storage, due to the diverse nature of the types of data implied by the different aspect of mobility; and integrating data from the private sector. Szeged is also sharing its mobility data with private companies.
To know more about this project, click here!
To know more about this project, click here!
COMMUTE’s goal is to alleviate heavy congestion leading to and from its airport’s business and industrial areas.
The City chose a collaborative governance model for the project, involving public and private mobility stakeholders.
This approach made it possible to share data between public and private stakeholders, enabling the partnership to carry out joint assessments, design strategies, deploy action plans, evaluate their impact and amend their strategies and action plans. The city reports that, although such collaboration is not easy, it is a necessary platform for the emergence of a shared vision.
As for several of the above city experiences with data, the city of Toulouse points to the need for a reliable data management system (in COMMUTE this takes the form of a digital platform) to support policy development and also the importance of anticipating the technical challenge of progressing from the mere gathering of heterogenous data sets to visualising data in a way that is useful for strategy development.
The COMMUTE strategy involves behaviour change more than building hard infrastructures (with the exception of autonomous shuttles). The project’s outreach component relies heavily on mobility data including static and real time data managed through the digital platform (journeys, flows, journey times, including third parties such as VéloToulouse etc.) as a basis for informing targeted schemes on car sharing, ride sharing, co-working spaces, teleworking, flexible timetables, carpooling, autonomous shuttles, etc.).
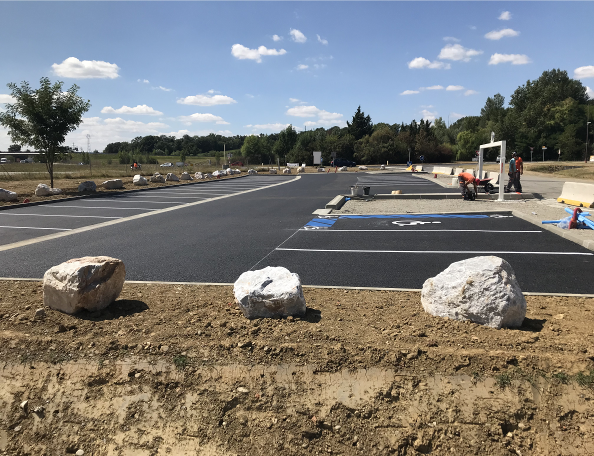
One of the core uses for data was in the development of KPIs, which required close collaboration with company human resources departments, which could provide the mobility data needed. This link between partnership and access to data is important, and COMMUTE highlights the importance of collaborative working to accessing the ‘data behind the data’. Toulouse also collects mobility data from citizens through various schemes (e.g., Je Participe, & VILAGIL integrated mobility project), while respecting GDPR rules. Toulouse also shares its data extensively with Google, Tom Tom, Waze, etc.
Toulouse, like several other UIA cities sees data management as a new profession and API a technical challenge. Toulouse recruited engineers specialised in the processing of big data and believes that the personnel responsible for analysing data must come from the transport professions as they must understand mobility issues to produce robust analyses.
To know more about this project, click here!
The importance of data
The UIA survey indicates that cities still count voyagers periodically using expensive conventional methods, although the data collected is not always of high quality (Lahti). With new modes emerging rapidly, this data can quickly become out-of-date. The Finnish national transport survey for example is conducted every 6 years. For the city of Ghent, the most important data source is a triennial resident survey. This allows the city to make modal split (demand) estimations, as well as estimate vehicle ownership and other indicators that are considered important for policy development. Ghent’s UIA TMaaS project used a co-creative approach exploiting data sourced and developed with private companies in response to the needs expressed by citizens.
Cities are beginning to incorporate automatic/real-time data to improve service provision and mobility policy but the survey feedback indicates that the task is not an easy one. Toulouse, for example, suggests that stakeholder consortia must first develop a reliable data management system, able to gather, process, and display data. In the same vein, several of the UIA surveyed cities cited managing large amounts of data in various formats as a key challenge.
The UIA survey indicates that cities still count voyagers periodically using expensive conventional methods, although the data collected is not always of high quality (Lahti). With new modes emerging rapidly, this data can quickly become out-of-date. The Finnish national transport survey for example is conducted every 6 years. For the city of Ghent, the most important data source is a triennial resident survey. This allows the city to make modal split (demand) estimations, as well as estimate vehicle ownership and other indicators that are considered important for policy development. Ghent’s UIA TMaaS project used a co-creative approach exploiting data sourced and developed with private companies in response to the needs expressed by citizens.
Cities are beginning to incorporate automatic/real-time data to improve service provision and mobility policy but the survey feedback indicates that the task is not an easy one. Toulouse, for example, suggests that stakeholder consortia must first develop a reliable data management system, able to gather, process, and display data. In the same vein, several of the UIA surveyed cities cited managing large amounts of data in various formats as a key challenge.
Categories of data use – mobility planning and management, advocacy, user participation, co-creation, and performance evaluation, etc.
Some of the specific uses of the new sources of data being exploited by the UIA cities include Lahti’s use of open data to offer free journey planner applications in Google’s Transit Trip Planner application. Ghent also developed a route planner for cyclists to guide them along recommended cycling routes because these routes were not identified by commercial services ‒ commercial route planners calculate the shortest routes, but not the better ones. Albertslund is building a sensor-based traffic model using traffic data collected by sensors in the city’s traffic lights. Albertslund is also making use of novel new technologies to obtain data, such as using drones to count traffic. Moreover, in Albertslund, the DOLL (Danish Outdoor Living Lab) is testing dynamic traffic detection, intelligent parking, and connected self-driving vehicles. Lahti’s entire bus fleet will be equipped with real-time tracking systems in the near future in order to share data and improve services. Szeged is using data to predict future Public Transport passenger flows and has installed new sensors in public transport with a view to optimising capacities and adapting public transport services, routes, and corridors.
The UIA cities are also involving citizens in mobility planning. Using their mobile devices, citizens are encouraged share not only their itineraries but also their views with city planners. Lahti, for example, is using a map-based tool, Maptionnaire, to gather opinions on the city’s cycle network and to add preferred new stations onto the map, and it is also being used to map daily routes and comfortable, uncomfortable, safe and unsafe places along residents’ daily routes. Involving citizens in developing the city’s transport system has also led to better cooperation on data sharing. In Lahti, for example, the Sustainable Urban Mobility Plan (SUMP) and Master Plan process use a co-creation approach that harnesses geospatial information generated by residents in a GIS system. In its CityMAP project, Lahti surveyed residents and found that some used private vehicles because they were unaware of sustainable transport options. It is such insights that can help cities to target investment on infrastructure/services where it can make a difference.
Policymakers cannot force behavioural change but outreach and persuasion can work. Szeged’s UIA project reveals the importance of public sector leadership and of its advocacy role in obtaining buy-in from business leaders, and, moreover, shows how key specific stakeholders are potentially in a position to influence individual transport decisions, not just of one person, but of entire workforces. This would be impossible without robust data, analytics and the ability to sell a vision. In a very similar vein, Toulouse shows how accessing certain useful mobility data would be difficult without the participation of employers.
A wide variety of data sets/specific uses are described in the survey feedback of which the above offers a rapid overview. The role of data reflects the multi-faceted nature of the goals assigned to mobility policy and the priorities of the cities surveyed, demonstrating the creativity of the cities surveyed – their extensive efforts at outreach ‒ and the potential that data offers policymakers ‒ from reducing CO2 to optimising traffic flows and from improving cycle routes to understanding a refuse vehicle’s contribution to congestion.
Some of the specific uses of the new sources of data being exploited by the UIA cities include Lahti’s use of open data to offer free journey planner applications in Google’s Transit Trip Planner application. Ghent also developed a route planner for cyclists to guide them along recommended cycling routes because these routes were not identified by commercial services ‒ commercial route planners calculate the shortest routes, but not the better ones. Albertslund is building a sensor-based traffic model using traffic data collected by sensors in the city’s traffic lights. Albertslund is also making use of novel new technologies to obtain data, such as using drones to count traffic. Moreover, in Albertslund, the DOLL (Danish Outdoor Living Lab) is testing dynamic traffic detection, intelligent parking, and connected self-driving vehicles. Lahti’s entire bus fleet will be equipped with real-time tracking systems in the near future in order to share data and improve services. Szeged is using data to predict future Public Transport passenger flows and has installed new sensors in public transport with a view to optimising capacities and adapting public transport services, routes, and corridors.
The UIA cities are also involving citizens in mobility planning. Using their mobile devices, citizens are encouraged share not only their itineraries but also their views with city planners. Lahti, for example, is using a map-based tool, Maptionnaire, to gather opinions on the city’s cycle network and to add preferred new stations onto the map, and it is also being used to map daily routes and comfortable, uncomfortable, safe and unsafe places along residents’ daily routes. Involving citizens in developing the city’s transport system has also led to better cooperation on data sharing. In Lahti, for example, the Sustainable Urban Mobility Plan (SUMP) and Master Plan process use a co-creation approach that harnesses geospatial information generated by residents in a GIS system. In its CityMAP project, Lahti surveyed residents and found that some used private vehicles because they were unaware of sustainable transport options. It is such insights that can help cities to target investment on infrastructure/services where it can make a difference.
Policymakers cannot force behavioural change but outreach and persuasion can work. Szeged’s UIA project reveals the importance of public sector leadership and of its advocacy role in obtaining buy-in from business leaders, and, moreover, shows how key specific stakeholders are potentially in a position to influence individual transport decisions, not just of one person, but of entire workforces. This would be impossible without robust data, analytics and the ability to sell a vision. In a very similar vein, Toulouse shows how accessing certain useful mobility data would be difficult without the participation of employers.
A wide variety of data sets/specific uses are described in the survey feedback of which the above offers a rapid overview. The role of data reflects the multi-faceted nature of the goals assigned to mobility policy and the priorities of the cities surveyed, demonstrating the creativity of the cities surveyed – their extensive efforts at outreach ‒ and the potential that data offers policymakers ‒ from reducing CO2 to optimising traffic flows and from improving cycle routes to understanding a refuse vehicle’s contribution to congestion.
Limits and challenges
Another important lesson from the feedback is that ‘data’ represents a multi-faceted challenge (volume, type, etc.). The specific challenges faced by the UIA cities are related to governance and technical issues. The question of data management should be seen in terms of the general challenge of implementing intelligent mobility. This requires sophisticated governance arrangements, at the functional or metropolitan scale, including cross-departmental working, the deployment of PPPs, and planning organisations able to exploit the potential of the data as will be discussed in Chapter 2. Data enables meaningful dialogue across organisations and departments. The need to harness data is therefore multi-faceted:
- The need to work on the metropolitan (functional area), cross-departmental, and/or cross-municipality scales with data enabling meaningful dialogue;
- The sheer volume of data (Ghent, Lahti), plus issues of managing/using data in different formats, the absence of metadata, which would make transfer easier (unclear purpose, difficult to synthesise);
- Designing a reliable data management system able to extract relevant information that can be visualised (Toulouse), including overcoming silo effects;
- Smaller cities may lack capacity (Szeged, Lahti report they lack the capacity they need); for the CEMR this may concern the legal, planning, or financial dimensions. The technical dimension should be added to this list;
- Some data remains ‘off the radar’ or may be ‘invisible’, for example, people not carrying a detectable device or who are unwilling to allow their data to be exploited, so conventional counting still matters;
- Gathering, storing, using, or sharing of certain types of data may be subject to regulation, privacy, cybersecurity, competition, or liability constraints. As indicated by Szeged and Lahti, the EU’s General Data Protection Regulation (GDPR) limits personal data collection, which limits the options of cities hoping to offer individualised services to the public. On the one hand, citizens can consent to companies collecting their personal data by opting in, which they could do when they sign up to GPS based navigation software applications for example. On the other hand, consent to use private and personal data is not often given for local authority data collection. The UIA survey picked up an interesting workaround to this. Szeged has patented a solution in the form of a software application which enables personalised information to be kept within sensors, only aggregated data can be extracted and analysed.
- Cities need to engage with and enter into collaborative arrangements (contracts, agreements) with third-party organisations, in compliance with security and privacy requirements, and opt for/develop a viable partnership framework, something all cities are having to deal with.
- Private organisations can supply various types of data, including about issues on the road network, e.g., MaaS-data, floating car data, employee mobility data, etc. Data sharing and collaboration are therefore critical because the next generation of mobility solutions and services will depend partly on data-sharing and joint solutions (Ghent, Szeged, Toulouse). However, it is important that local governments remain accountable, that they set the rules, e.g., on data anonymity, etc. It is also paramount for local governments to be able to monitor and evaluate results, and to be able to adjust the system by ensuring it has full access to the data and the analytics.
Another important lesson from the feedback is that ‘data’ represents a multi-faceted challenge (volume, type, etc.). The specific challenges faced by the UIA cities are related to governance and technical issues. The question of data management should be seen in terms of the general challenge of implementing intelligent mobility. This requires sophisticated governance arrangements, at the functional or metropolitan scale, including cross-departmental working, the deployment of PPPs, and planning organisations able to exploit the potential of the data as will be discussed in Chapter 2. Data enables meaningful dialogue across organisations and departments. The need to harness data is therefore multi-faceted:
- The need to work on the metropolitan (functional area), cross-departmental, and/or cross-municipality scales with data enabling meaningful dialogue;
- The sheer volume of data (Ghent, Lahti), plus issues of managing/using data in different formats, the absence of metadata, which would make transfer easier (unclear purpose, difficult to synthesise);
- Designing a reliable data management system able to extract relevant information that can be visualised (Toulouse), including overcoming silo effects;
- Smaller cities may lack capacity (Szeged, Lahti report they lack the capacity they need); for the CEMR this may concern the legal, planning, or financial dimensions. The technical dimension should be added to this list;
- Some data remains ‘off the radar’ or may be ‘invisible’, for example, people not carrying a detectable device or who are unwilling to allow their data to be exploited, so conventional counting still matters;
- Gathering, storing, using, or sharing of certain types of data may be subject to regulation, privacy, cybersecurity, competition, or liability constraints. As indicated by Szeged and Lahti, the EU’s General Data Protection Regulation (GDPR) limits personal data collection, which limits the options of cities hoping to offer individualised services to the public. On the one hand, citizens can consent to companies collecting their personal data by opting in, which they could do when they sign up to GPS based navigation software applications for example. On the other hand, consent to use private and personal data is not often given for local authority data collection. The UIA survey picked up an interesting workaround to this. Szeged has patented a solution in the form of a software application which enables personalised information to be kept within sensors, only aggregated data can be extracted and analysed.
- Cities need to engage with and enter into collaborative arrangements (contracts, agreements) with third-party organisations, in compliance with security and privacy requirements, and opt for/develop a viable partnership framework, something all cities are having to deal with.
- Private organisations can supply various types of data, including about issues on the road network, e.g., MaaS-data, floating car data, employee mobility data, etc. Data sharing and collaboration are therefore critical because the next generation of mobility solutions and services will depend partly on data-sharing and joint solutions (Ghent, Szeged, Toulouse). However, it is important that local governments remain accountable, that they set the rules, e.g., on data anonymity, etc. It is also paramount for local governments to be able to monitor and evaluate results, and to be able to adjust the system by ensuring it has full access to the data and the analytics.
City mobility: management challenges in light of the growing centrality of data
The centrality of data for delivering intelligent mobility leads to the question of governance capacity. The CEMR and the Urban Agenda for the EU see governance capacity in terms of ‘city and regions with a lead role, supported by national governments and Europe with appropriate legislative frameworks and adequate funding’. The CEMR/Urban Agenda argue that mobility planning requires well-resourced project teams with a range of skills, ranging from legal (policymaking) and planning competencies. The technical dimension of data management must be added to this.
Whether considering an individual city or partnership-based mobility project, data has a central role. And from this technical perspective, the challenge is not just data (big data) and its multiple uses/challenges. Policymakers are faced with a suite of new and disruptive technologies including the electrification of vehicles, new powertrains, MaaS, and connected and autonomous vehicles[1], and so on.
It is worth reiterating that cities are spaces where multiple trends are merging; they are at the confluence of rapid technological change (EVs, digital revolution), urgent regulatory change (climate, pollution) and societal change (lifestyles).
The UIA cities and their stakeholders, including private businesses and citizens, are today collaborating and data has a central role in the initiatives surveyed. Data is being seen as the ‘new oil’ in the ‘nervous system’ of future sustainable urban mobility systems. This brings us to the implications of this crucial and growing role for data.
[1] KPMG Mobility 2030: Transforming the mobility landscape, 2019.
The centrality of data for delivering intelligent mobility leads to the question of governance capacity. The CEMR and the Urban Agenda for the EU see governance capacity in terms of ‘city and regions with a lead role, supported by national governments and Europe with appropriate legislative frameworks and adequate funding’. The CEMR/Urban Agenda argue that mobility planning requires well-resourced project teams with a range of skills, ranging from legal (policymaking) and planning competencies. The technical dimension of data management must be added to this.
Whether considering an individual city or partnership-based mobility project, data has a central role. And from this technical perspective, the challenge is not just data (big data) and its multiple uses/challenges. Policymakers are faced with a suite of new and disruptive technologies including the electrification of vehicles, new powertrains, MaaS, and connected and autonomous vehicles[1], and so on.
It is worth reiterating that cities are spaces where multiple trends are merging; they are at the confluence of rapid technological change (EVs, digital revolution), urgent regulatory change (climate, pollution) and societal change (lifestyles).
The UIA cities and their stakeholders, including private businesses and citizens, are today collaborating and data has a central role in the initiatives surveyed. Data is being seen as the ‘new oil’ in the ‘nervous system’ of future sustainable urban mobility systems. This brings us to the implications of this crucial and growing role for data.
[1] KPMG Mobility 2030: Transforming the mobility landscape, 2019.
Towards civil servant 2.0 and (technical) governance capacity building for data management
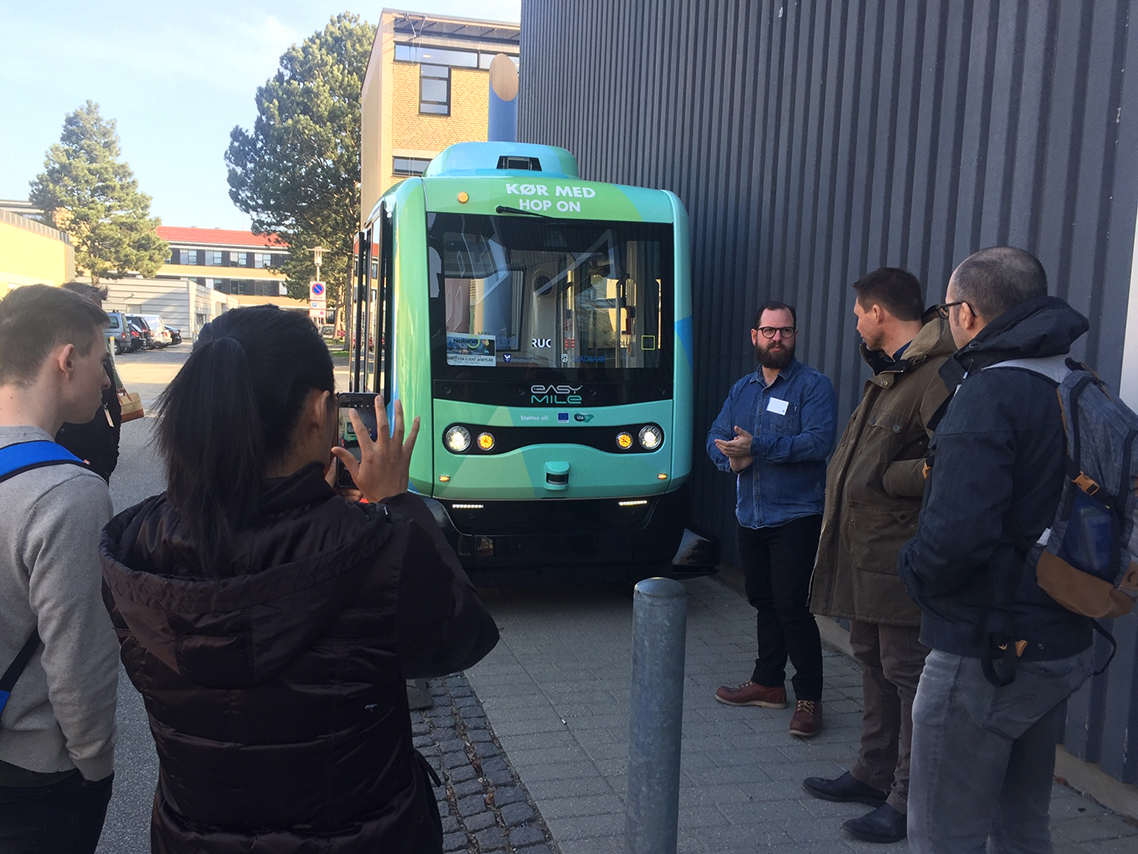
Knowing that data management is a prerequisite for the new mobility governance, an obvious question arises. Are cities ready to manage data effectively and what should be done if not? This is primarily question for cities, their partnerships, and mobility project teams. It is also a question that national and European policymakers need to fully take into account.
The main component in this data readiness issue is personnel. In this regard, new professions are emerging. One is the data analyst. Data analytics is specialised and requires specific skill-sets; the traditional skill of civil servants may be inadequate. Experts consulted as part of this UIA survey noted for example how Toulouse recruited engineers specialised in data processing. This suggests that it may be necessary to raise awareness and train city planners and public transport service providers on the promise and challenges of data and the contribution data analytics can make to meeting mobility challenges. Mobility planning teams need to possess a wide range of expertise in both transport planning and big data analysis, so the skill-sets required are hybrid. Cities or wider partnership-based projects need to build up in-house capacity although there is an option to outsource. Most cities acknowledge the need to have resources dedicated to data collection, data analysis, and visualisation. However, job descriptions, recruitment plans etc. are slow to emerge and missions are being allocated to external experts/companies on ad hoc basis. Again, the answer to ‘which is the better approach?’ depends on how a city wishes to deliver its vision and on its resources.
The survey shows that cities are engaging with big data analysis ‘in-house’ (such as Lahti, which, like Albertslund, developed a centralised data collection platform), and within partnerships with firms (such as in Toulouse, which has made extensive use of PPPs; COMMUTE has its own digital platform), including merging mobility data with other sources of data (such in Ghent’s TMaaS). Nevertheless, when it comes to real time/big data research indicates that cities can lack knowledge and experience including with regard to data management capabilities, and they can also lack the capacity to reach out to the public. An up-to-date understanding of the potential digital tools and technologies is fundamental to surveying user satisfaction with sustainable mobility solutions. A recent study on the digital preparedness in urban sustainable transport conducted by UITP (2019) found that 62% of transport authority respondents did not have enough employees with the necessary digital expertise.
This brings the discussion back to capacity building. The need for appropriate support to cities from the national and European levels and the importance of partnerships is clear, and it is also useful to reflect on the policy challenge and the stakes involved, and specifically the immediacy of the need to reboot cities for a post-oil mobility model, and the magnitude of the task(s). In this context, the question facing city planners is where to place the curser between pragmatism and ambition. Take mobility data platforms for example. Veeneman et al (2018) studied the success factors in the creation of mobility platforms that ‘combine a wide variety of data sources to support the creation of an overview of network status and flows (historical, current, and future), and allow for use of that information from real-time travel planning to long-term infrastructure planning’. While such platforms are only one ingredient in what cities are aiming to achieve, the authors’ findings, based on a study of 13 cities, are useful. A first lesson, that the greater the technical ambition of the platform, the greater the capacity challenge involved, is obvious. A second lesson however is food for thought. It is that the governance capacity may sometimes be a given, in other words capacity may not be easy to change. Veeneman suggests that, for this reason, the level of ambition of a platform project should be tailored to existing capacity (although as Ghent and Toulouse and other cities show, a PPP can in effect increase capacity).
On the question of ambition, the CEMR/Urban Agenda’s recommends that national governments, with EU support, should ensure that cities or partnerships have the legal, planning, and financial resources they need. From the UIA survey, we can say that cities will be as ambitious as they are able to be within the frameworks established by national governments. The UIA city experience also suggests that technical expertise should be added to the CEMR/Urban agenda resource categories.
Knowing that data management is a prerequisite for the new mobility governance, an obvious question arises. Are cities ready to manage data effectively and what should be done if not? This is primarily question for cities, their partnerships, and mobility project teams. It is also a question that national and European policymakers need to fully take into account.
The main component in this data readiness issue is personnel. In this regard, new professions are emerging. One is the data analyst. Data analytics is specialised and requires specific skill-sets; the traditional skill of civil servants may be inadequate. Experts consulted as part of this UIA survey noted for example how Toulouse recruited engineers specialised in data processing. This suggests that it may be necessary to raise awareness and train city planners and public transport service providers on the promise and challenges of data and the contribution data analytics can make to meeting mobility challenges. Mobility planning teams need to possess a wide range of expertise in both transport planning and big data analysis, so the skill-sets required are hybrid. Cities or wider partnership-based projects need to build up in-house capacity although there is an option to outsource. Most cities acknowledge the need to have resources dedicated to data collection, data analysis, and visualisation. However, job descriptions, recruitment plans etc. are slow to emerge and missions are being allocated to external experts/companies on ad hoc basis. Again, the answer to ‘which is the better approach?’ depends on how a city wishes to deliver its vision and on its resources.
The survey shows that cities are engaging with big data analysis ‘in-house’ (such as Lahti, which, like Albertslund, developed a centralised data collection platform), and within partnerships with firms (such as in Toulouse, which has made extensive use of PPPs; COMMUTE has its own digital platform), including merging mobility data with other sources of data (such in Ghent’s TMaaS). Nevertheless, when it comes to real time/big data research indicates that cities can lack knowledge and experience including with regard to data management capabilities, and they can also lack the capacity to reach out to the public. An up-to-date understanding of the potential digital tools and technologies is fundamental to surveying user satisfaction with sustainable mobility solutions. A recent study on the digital preparedness in urban sustainable transport conducted by UITP (2019) found that 62% of transport authority respondents did not have enough employees with the necessary digital expertise.
This brings the discussion back to capacity building. The need for appropriate support to cities from the national and European levels and the importance of partnerships is clear, and it is also useful to reflect on the policy challenge and the stakes involved, and specifically the immediacy of the need to reboot cities for a post-oil mobility model, and the magnitude of the task(s). In this context, the question facing city planners is where to place the curser between pragmatism and ambition. Take mobility data platforms for example. Veeneman et al (2018) studied the success factors in the creation of mobility platforms that ‘combine a wide variety of data sources to support the creation of an overview of network status and flows (historical, current, and future), and allow for use of that information from real-time travel planning to long-term infrastructure planning’. While such platforms are only one ingredient in what cities are aiming to achieve, the authors’ findings, based on a study of 13 cities, are useful. A first lesson, that the greater the technical ambition of the platform, the greater the capacity challenge involved, is obvious. A second lesson however is food for thought. It is that the governance capacity may sometimes be a given, in other words capacity may not be easy to change. Veeneman suggests that, for this reason, the level of ambition of a platform project should be tailored to existing capacity (although as Ghent and Toulouse and other cities show, a PPP can in effect increase capacity).
On the question of ambition, the CEMR/Urban Agenda’s recommends that national governments, with EU support, should ensure that cities or partnerships have the legal, planning, and financial resources they need. From the UIA survey, we can say that cities will be as ambitious as they are able to be within the frameworks established by national governments. The UIA city experience also suggests that technical expertise should be added to the CEMR/Urban agenda resource categories.
[1] Urban Mobility Daily. Why Open Data is Critical to the Future of MaaS, Sandra Witzel, 23/09/2020.
[2] McKinsey & Company. Public-private collaborations for urban mobility. Shannon Bouton, Non 1, 2017.
[3] The Intelligent Mobility Experience, Micromobility, The first and last mile: the problem and the solutions.
[4] CATAPULT, Article: Intelligent Mobility – driving a £1.4 trillion market.
[5] The Catapult Data Revolution, Investigation into the data required to support and drive intelligent mobility, CATAPULT, March 2015.
[6] FRENCH GOVT: La loi d'orientation des mobilités, published in the Journal officiel, 26 December, 2019 / UK GOVT Data and digital systems for UK transport: change and its implications, Dr Caitlin D Cottrill, UK Govt Office for Science, Future of Mobility, Evidence Review, Dec 2018.
[7] France: invest in new infrastructures and in parallel to ‘enable’ new transport services, with data sharing and open data at the heart of the approach. which seeks to open up public mobility data and imposes obligations on private sector providers to share data.
[8] The Open Data Institute, Briefing Paper, The UK must support data sharing and open data in transport, April 12, 2017.
[9] International Road Federation, Data Sharing for Urban Mobility, July 2020. News article.
[10] Smart Mobility and Services, Expert group report for the EU Commission, 2017. EUR KI-01-17-928-EN-N.
[11] SMILE B2G data sharing, European research project, Data policy and Innovation team.
[12] CIVITAS Insight N°18, August 2016.
Concluding remarks and recommendations on the importance of data in mobility are presented in the final chapter of this report.
About this resource
#SCEWC24 treasure hunt:
Reach the next level --> explore this page and find the button "Climate Adaptation", hidden in the "Green" part.
Then, you have to find an "Urban practice" located in Paris.
The Urban Innovative Actions (UIA) is a European Union initiative that provided funding to urban areas across Europe to test new and unproven solutions to urban challenges. The initiative had a total ERDF budget of €372 million for 2014-2020.
Similar content
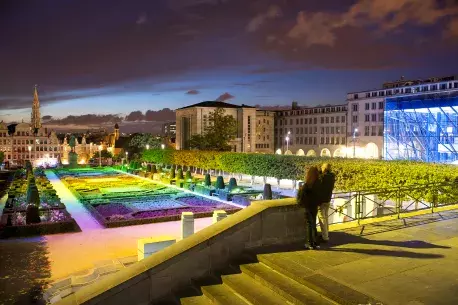
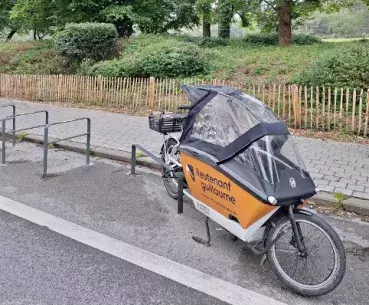
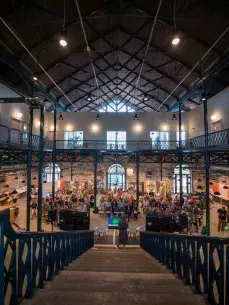